[Quipu Issue Paper] Genome Annotation Ⅵ - Functional annotation(비교유전체 분석)
- Posted at 2010/03/24 10:32
- Filed under 생물정보
1. Assembly
2. Variation study
3. Expression study
4. Epigenomics
5. Genome Annotation
6. Next Generation Bioinformatics
7. Data Management for web 2.0 Era
8. Semantic Network for Integrated Biology Data
9. Gene Network Discovery by Text-mining
10. Centralization for High-throughput Data Analysis
이번 연재에서는 서로 다른 종간의 상응하는 유전자 조합 및 구성을 분석하여 진화론적인 유연관계를 밝히는 비교유전체 분석에 대해 알아보겠습니다.
B. 비교유전체 분석
서로 다른 종간의 상응하는 유전자 조합 및 구성을 분석하여 진화론적인 유연관계를 밝히는 비교유전체 분석의 가장 기본적인 분석은 ortholog 분석이다. Ortholog란 한 개체가 진화되어 여러 종으로 분화되었을 때, 유전자의 기능이 그대로 보존되어 서로 유사한 기능을 수행하는 것을 의미한다. 미생물의 경우 단순 ortholog 분석에서 확장되어 오페론 단위의 유전자 그룹을 유전체 상의 위치 정보와 함께 분석하는 Positional linkage 방법이 매우 정확하게 분석되고 있다(그림 10).
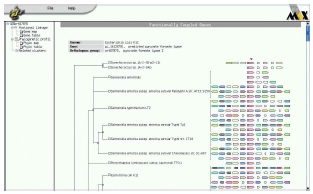
진화론적인 연관관계 분석 시 이웃하고 있는 유전자의 배열과 조성을 통해 종간 변이 단계를 분석하게 된다. 좀 더 나아가 특정 ortholog 그룹의 유전자들의 존재 유무를 다수의 종에서 분석하여 프로파일링을 수행하고 그 프로파일을 이용하여 유연관계를 밝히는 phylogenetic 프로파일링 기법도 최근 매우 정확히 유전자의 기능을 예측하는 알고리즘으로 알려지고 있다. 비슷한 단백질 프로파일을 갖는 것은 비슷한 기능을 갖는다는 의미를 보여주기 때문에 유전자 기능 분석 시 이용되고 있다(그림 11).
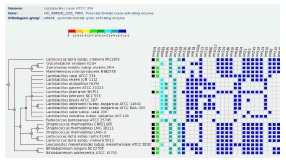
다음 연재에서는 수학적 알고리즘에 의한 유전자 예측으로 생각할 수 없었던 예외적인 사항이 많이 발생한다. 이러한 부분을 실제 유전자의 구조를 하나씩 살펴가며 수정 작업을 거치는 Professional Curation에 대해 알아보겠습니다. 많은 관심 부탁드립니다.
1. Lowe, T.M. and Eddy, S.R. (1997) tRNAscan-SE: a program for improved detection of transfer RNA genes in genomic sequence. Nucleic Acids Res. 25, 955-964.
2. Lewis SE, et al. (2002). Apollo: a sequence annotation editor. Genome Biology. 12, research0082
3. Noh SJ, Lee K, Paik H, Hur CG. (2006) TISA: tissue-specific alternative splicing in human and mouse genes. DNA Res. 13, 229-243
4. Stanke M, Schoffmann O, Morgenstern B, Waack S. (2006) Gene prediction in eukaryotes with a generalized hidden Markov model that uses hints from external
sources. BMC Bioinformatics. 7, 62.
5. Burge, C. and Karlin, S. (1997) Prediction of complete gene structures in human genomic DNA. J. Mol. Biol. 268, 78-94.
6. Salamov AA, Solovyev VV. (2000) Ab initio gene finding in Drosophila genomic DNA. Genome Res. 10, 516–522.
7. Majoros, W.H., Pertea, M., and Salzberg, S.L. TigrScan and GlimmerHMM: two open-source ab initio eukaryotic gene-finders Bioinformatics 20, 2878-2879.
8. G. Parra, E. Blanco, and R. Guigó, (2000) Geneid in Drosophila Genome Research 4, 511-515.
9. Haas BJ, Salzberg SL, Zhu W, Pertea M, Allen JE, Orvis J, White O, Buell CR, Wortman JR. (2008) Automated eukaryotic gene structure annotation using
EVidenceModeler and the Program to Assemble Spliced Alignments. Genome Biology 9, R7
10. Korf I. (2004) Gene finding in novel genomes. BMC Bioinformatics. 5, 59.
11. Kan, Z., Rouchka, E.C., Gish, W., and States, D. 2001, Gene structure prediction and AS analysis using genomically aligned ESTs, Genome Res. 11, 889–900.
12. Eyras, E., Caccamo, M., Curwen, V., and Clamp, M. 2004, ESTGenes: AS from ESTs in Ensembl, Genome Res. 14, 976–987.
13. Kent, W.J. 2002, BLAT-The BLAST-Like Alignment Tool, Genome Res. 12, 565–664.
14. Florea, L., Hartzell, G., Zhang, Z., Rubin, G.M., Miller, W. 1998, Computer program for aligning a cDNA sequence with a genomic DNA sequence, Genome Res. 8,
967–974.
15. Huang X, Adams MD, Zhou H, Kerlavage AR. (1997) A tool for analyzing and annotating genomic sequences. Genomics. 46, 37–45.
16. Wu TD, Watanabe CK. (2005) GMAP: a genomic mapping and alignment program for mRNA and EST sequences. Bioinformatics. 21, 1859–1875.
17. Birney E, Clamp M, Durbin R. (2004) GeneWise and Genomewise. Genome Res. 14, 988–995.
Posted by 人Co
- Tag
- Curation, Genome Annotation, insilicogen, NGS, operon, Ortholog, phylogenetic, Positional linkage, 인실리코젠, 진화
- Response
- No Trackback , No Comment
- RSS :
- https://post-blog.insilicogen.com/blog/rss/response/62